https://github.com/bmcfee/librosa
https://github.com/jamiebullock/LibXtract/
http://web.mit.edu/music21/
https://wiki.python.org/moin/PythonInMusic
https://github.com/danstowell/smacpy
simple-minded audio classifier in python (using MFCC and GMM)
Music Genre Classification
Model AI Assignment
EAAI-12: Third Symposium on Educational Advances in Artificial Intelligence
Toronto, Ontario, Canada
July 23-24, 2012
Douglas Turnbull
Department of Computer Science
Ithaca College
dturnbull@ithaca.edu
Summary | Music Genre Classification: students explore the engaging topic of content-based music genre classification while developing practical machine learning skills |
Topics | Main Focus: supervised learning, bag-of-feature-vector representation, the Gaussian classifier, k-Nearest Neighbor classifier, cross-validation, confusion matrix |
Audience | Undergraduate students in an introductory course on artificial intelligence, machine learning, or information retrieval |
Difficulty | Students must be familiar with file input/output, basic probability and statistics (e.g., multi-variate Gaussian distribution), and have a basic understanding of western popular music (e.g., rock vs. techno genres). |
Strengths | Music is interesting. Most college-aged students have an iPod, use Pandora, go to live shows, share music with their friends, etc. To this end content-based music analysis is a fun, engaging, and relevant topic for just about every student. Using music, this assignment motivates a number of important machine learning topics that are useful for computer vision, recommender systems, multimedia information retrieval, and data mining. |
Weaknesses | Requires a bit of file management to get started. However, sample Matlab code has been provided to help with file I/O. |
Dependencies | This lab can be done using any programming languages though Matlab or Python (with the Numpy library) is recommended. |
Variants | Data Mining Competition: can serve as a "bake-off" assignment where students can propose and implement their own classification approach. Undergraduate Research Project: music analysis is a hot research topic and students are often interested in learning more about it. Related projects might include using digital signal processing to directly analyze audio content, text-mining music blogs, collecting music information extracted from popular social networks (last.fm, Facebook), and building music recommendation applications (iTunes Genius, Pandora). |
Index
- Overview
- Objectives
- Background Reading
- Details
- Discussion Questions
- Possible Extensions
- Final Remarks
Overview
In this lab, you will learn how a computer can automatically classify songs by genre through the analysis of the audio content. We provide a data set consisting of 150 songs where each song can be associated with one of the six genres.Each song is represented by a bag-of-feature-vectors. Each 12-dimensional feature vector represents the timbre, or "color", of the sound for a short (less than one second) segment of audio data. If we think about each feature vector as being a point in a 12-dimensional timbre space, then we can think of a song being a cloud of points in this same timbre space. Furthermore, we can think of many songs from a particular genre as occupying a region in this space. We will use a multivariate Gaussian probability distribution to model the occupying region of timbre for each genre.
When we are given a new unclassified song, we calculate the probability of the song's bag-of-audio-feature-vectors under each of the six Gaussian genre models. We then predict that the genre with the highest probability. We can evaluate the accuracy of our Gaussian classifier by comparing how often the predicted genre matches the true genre for songs that were not originally used to create the Gaussian genre models.
Objectives
This lab approachs music genre classification as a standard supervised learning problem. Specifically, students will learn about:- important supervised learning concepts (training, evaluation, cross-validation)
- the bag-of-feature-vector representation
- a Gaussian classifier
- a k-Nearest Neighbor classifier
Background Reading
Many textbooks provide a general background material on supervised learning (e.g., Russell & Norvig's AI: A Modern Approach and Duda, Hart & Stork's Pattern Classifiation). In addition, lots of information about supervised machine learning can be found on the web (e.g., Wikipedia).For this lab in particular, below is a list of three good references related to content-based music genre classification:
- Echo Nest Analyze Documentation - provides a background on how the timbre-based audio features are computed using digital signal processing. It also provides information about other available audio features related to rhythm, key, tempo, harmony and loudness.
- Music Genre Classification of Audio Signals by Tzanetakis & Cook (2002)- a seminal work on the music genre classification problem. This paper is accessible to undergraduate AI students and provides them experience reading scholarly works.
- Exploring Automatic Music Annotation with Acoustically-Objective Tags by Tingle, Kim, & Turnbull (2010) - a more recent music classification paper that connects the Tzanetakis paper with the Echo Nest audio features. This paper also serves as an example of how an undergraduate student researcher (Tingle) can make a contribution to the field of music information retrieval.
In addition, the Music Information Retrieval Evaluation eXchange (MIREX) is an annual evaluation for various music information retrieval tasks. Each year, music classification is one of the most popular tasks and you can read about the best performing systems. If you develop a solid classification system, consider submitting it to MIREX next year!
Details
This lab can be done using any programming language, although Python (with the Numpy library) and Matlab have been found to work well.Step 1: Load up the data
In the data/ directory, you will find six subdirectories for six genres of music: classical, country, jazz, pop, rock, and techno. Each folder contains 25 data files for 25 songs that are associated with the specific genre. The relationship between a song and a genre is determined by social tagging and obtained using the Last.fm API.The files are formatted as follows:
# Perhaps Love - John Denver
0.0,171.13,9.469,-28.48,57.491,-50.067,14.833,5.359,-27.228,0.973,-10.64,-7.228
26.049,-27.426,-56.109,-95.41,-40.974,99.266,-5.217,-18.986,-27.03,59.921,60.989,-4.059
35.338,5.255,-40.244,-14.309,32.12,30.625,9.415,-8.023,-27.699,-45.148,23.829,20.7
...
where the first line starts with a # symbol followed by the song name and artist. You can hear samples of most songs using Spotify, the Apple iTunes Store, last.fm, YouTube or any other music hosting site. Each following line consists of 12 decimal numbers that together represent the audio content for a short, stable segment of music. You can think of these numbers as a 12-dimensional representation of the various frequencies that make up a musical note or chord in the song. There are between about 300 to about 1300 segments per song. This number depends on both the length of the song (i.e., longer songs tend to have more segments), but also on the beat (i.e., fast vs. slow tempo) and timbre (e.g., noisy vs. minimalist) of the music. Below is a visual representation of how we can represent a song as a bag-of-feature-vectors:
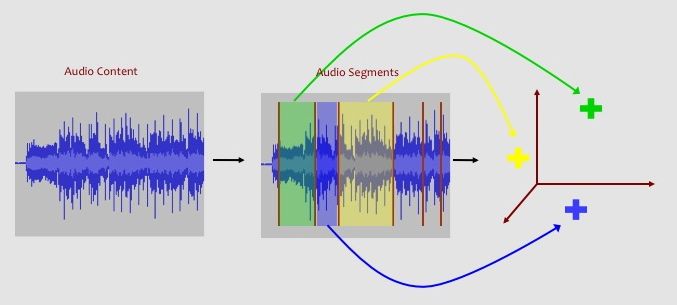
Before Moving On: your program should load the audio feature matrices and associated metadata from the 150 data files.
Step 2: Learning a Gaussian Distribution for each Genre
For each of the 6 genres, you will want to randomly select 20 of the 25 songs to serve as a training set. (We will use the other 5 songs to evaluate the system in step 3.)For each genre, you will need to calculate a 12-dimensional mean vector and a 12x12-dimensional covariance matrix. These 12+144 numbers fully describe our probabilistic model for the genre. (Note: If some genre were more common than other genres, we would have to store this additional information in the model as well. This is sometimes called the prior probability of a genre.) Below is a visual representation of how we can model the audio content from a set of songs using a Gaussian distribution:
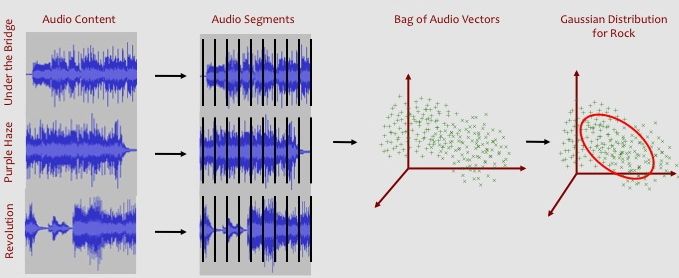
Note: Most programming languages (Matlab, Python-Numpy) have math libraries that provide useful mean, covariance, matrix transpose and matrix inverse functions. See the programming language documentation for details. To code these function from scratch, refer to an AI textbook, a statistics textbook, or Wikipedia for standard definitions of each concept.
Before Moving On: you should have one mean vector (12-dimensional), one covariance matrix (12x12-dimensional), and one inverse covariance matrix (12x12-dimensional) for each of the 6 genres.
Step 3: Predicting the Genre of a Song
We will combine the 5 remaining songs for each of the 6 genres into a 30-song evaluation set. For each of these songs, we will calculate the probability of the song's bag-of-audio-feature-vectors for each of the 6 Gaussian distributions that we trained in the previous step. More specifically, we will calculate the average unnormalized negative log likelihood (average-UNLL) of a song given a Gaussian distribution. While this might sound like a mouthful, we just want to find out how well the new song fits with each of the genre models that we learned from the training data. Below is a visual representation what we mean when we say "how good a new song fits a model":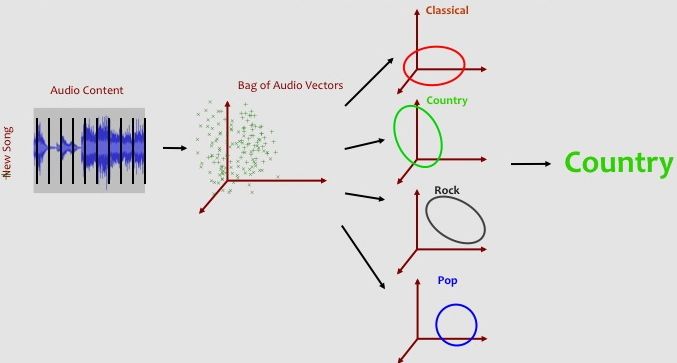
UNLL = (x - mean_genre) * inverse(cov_genre) * transpose(x - mean_genre)
where x is the 1x12 audio feature vector, mean_genre is the 1x12 mean vector calculate in step 2, and inverse(cov_genre) is the 12x12 inverse of the covariance matrix also calculated in step 2. Finally, we then find the average UNLL for all of the audio vectors of the song.
Once we have calculated the average-UNLL for a song under each of the 6 Gaussian genre models, we simply predict the genre associated with the smallest average-UNLL value. If the true genre matches the predicted genre, we have accurately classified the song.
Before Moving On: You should calculate the average-UNLL for each of the 30 test set songs and each of the 6 genre models.
Step 4: Evaluating Our Gaussian Classifiers
The accuracy of our system is the percentage of songs that are accurately classified by our system. For the given data set, you should get about 55% accuracy. While this might seem low, random guessing would get us about 16% accuracy (i.e., 1/6 chance). Note that your performance might differ based on how we randomly split our data into training and evaluation data sets. If you were to re-run this experiment a bunch of times, you would see that the performance bounces around a bit. However, if we take the average accuracy for a number of random splits, we would be able to better estimate the true accuracy of our system. This process is called random cross-validation.We can further explore the data by noting which genres are relatively easy to classify and which pairs of genres are often confused with one another. Try filling out the 6x6 confusion matrix to help you visualize this information better. One axis of this matrix represents the true genre label while the other axis represents the predicted label. The diagonal cells represent accurate predictions while the off-diagonal cells indicated which pairs of genres are likely to be confused with one another.
To probe deeper into the results, you can look at individual mistakes made by our classification system. For example, you may find that the system predicted a pop song as being a techno song. However, upon closer inspection, the specific pop song may have a strong synthesized beat that is characteristic of most techno songs. This kind of qualitative analysis can provide us with a better understanding of how music genres are related to one another.
Before Moving On: You should calculate the classification accuracy and confusion matrix for the Gaussian classifier.
(Optional) Step 5: Comparing Against a k-Nearest Neighbor Classifier
There are many algorithms that we can use to classify music by genre. One common and easy-to-implement classifier is call the k-Nearest Neighbor (kNN) classifier. You can implement this classifier and see how its performance compares to the Gaussian classifier. Here is how it works:First, instead of learning a Gaussian Distribution for each genre, estimate the parameters (mean vector, covariance matrix) for each song in the training set. This will produce 120 Gaussian distributions.
Second, calculate the average-UNLL between each of the 30 test set songs and each of the 120 training set Gaussian distribution. This will result in a 30x120 dimensional matrix.
Third, pick a small odd number k (e.g., k = 1, 3, or 5). Then, for each test set song, find the "k nearest neighbor" songs in the training set. These are the k songs in the training set with the smallest average-UNLL.
Fourth, use the genre labels for these nearest neighbor to predict the label of the test set song. For example, if the the five nearest neigbor of a test set song are associated with the genres [pop, rock, rock, jazz, rock], we would predict the song is a rock song because three of the nearest neighbors are rock. We can break ties randomly or use some other heuristic (e.g., closest neighbor wins).
Once you have predicted a genre for each of the test set songs using the kNN classifier, you can evaluate the classifier using accuracy as described in step 5.
Discussion Questions
- What assumptions about the data do we make when we model the date using a Gaussian distribition?
- When do you expect that a Gaussian will work well and when do you think it will not work well?
- What values of k work best for the kNN classifier?
- Based on your results from this assignment, which classifier (Gaussian or kNN) works best for the task of Music Genre Classification?
- Why do you think each classifier performed as well (or as poorly ) as it did?
- Can you think of ways that you can modify the classifiers so that you might improve performance?
Possible Extensions
This lab is designed to help you build a classification system from scratch. There are many ways to extend this work:- Explore Your Own Music: Using the Echo Nest API, you can upload your own music. Also, if you want to check out a huge data set of music, you should check out the Million Song Dataset.
- Try Additional Audio Features: You can also use the Echo Nest API to get additional audio features that relate to tempo, key, mode, harmony, etc. Note that the file names in the data/ directory are Echo Nest Track IDs so you can obtain these features without owning a copy of the individual audio tracks. Alternatively, you use digital signal processing to calculate your own audio features.
- Use Social Information: While this lab focused on content-based music classification, you can collect social information (blog entries, rating data, social tags) from a number of public APIs (Echo Nest, Last.fm, Facebook, etc.)
- Develop Alternative Classifiers: In this lab, we focused on a Gaussian classifier and a k-nearest neighbor classifier. However, this is just one of many classification systems. Other common classifiers include a support vector machine (SVM), a Gaussian mixture model(GMM), or a hidden Markov model (HMM). Better yet, invent your own classification algorithm.
Final Remarks
I hope that you enjoyed building a content-based music classifier. Music information retrieval is a young and active research area. Once you have developed your ideas, consider submitting your work to theISMIR conference or entering your system in the MIREX evaluation exchange.ESSENTIA: an open source library for audio analysis
Authors: Dmitry Bogdanov, Nicolas Wack, Emilia Gómez, Sankalp Gulati, Perfecto Herrera, Oscar Mayor, Gerard Roma, Justin Salamon, Jose Zapata, Xavier Serra, Music Technology Group, Universitat Pompeu Fabra
More information: http://essentia.upf.edu
Over the last decade, audio analysis has become a field of active research in academic and engineering worlds. It refers to the extraction of information and meaning from audio signals for analysis, classification, storage, retrieval, and synthesis, among other tasks. Related research topics challange understanding and modeling of sound and music, and develop methods and technologies that can be used to process audio in order to extract acoustically and musically relevant data and make use of this information. Audio analysis techniques are instrumental in the development of new audio-related products and services, because these techniques allow novel ways of interaction with sound and music.

Essentia is an open-source C++ library for audio analysis and audio-based music information
retrieval released under the Affero GPLv3 license (also available under proprietary license upon request). It contains an extensive collection of reusable algorithms which implement audio input/output functionality, standard digital signal processing blocks, statistical characterization of data, and a large set of spectral, temporal, tonal and high-level music descriptors that can be computed from audio. In addition, Essentia can be complemented with Gaia, a C++ library with python bindings which allows searching in a descriptor space using different similarity measures and classifying the results of audio analysis (same license terms apply). Gaia can be used to generate classification models that Essentia can use to compute high-level description of music.
retrieval released under the Affero GPLv3 license (also available under proprietary license upon request). It contains an extensive collection of reusable algorithms which implement audio input/output functionality, standard digital signal processing blocks, statistical characterization of data, and a large set of spectral, temporal, tonal and high-level music descriptors that can be computed from audio. In addition, Essentia can be complemented with Gaia, a C++ library with python bindings which allows searching in a descriptor space using different similarity measures and classifying the results of audio analysis (same license terms apply). Gaia can be used to generate classification models that Essentia can use to compute high-level description of music.
Essentia is not a framework, but rather a collection of algorithms wrapped in a library. It doesn’t enforce common high-level logic for descriptor computation (so you aren’t locked into a certain way of doing things). It rather focuses on the robustness, performance and optimality of the provided algorithms, as well as ease of use. The flow of the analysis is decided and implemented by the user, while Essentia is taking care of the implementation details of the algorithms being used. A number of examples are provided with the library, however they should not be considered as the only correct way of doing things.
The library includes Python bindings as well as a number of predefined executable extractors for the available music descriptors, which facilitates its use for fast prototyping and allows setting up research experiments very rapidly. The extractors cover a number of common use-cases for researchers, for example, computing all available music descriptors for an audio track, extracting only spectral, rhythmic, or tonal descriptors, computing predominant melody and beat positions, and returning the results in yaml/json data formats. Furthermore, it includes a Vamp plugin to be used for visualization of music descriptors using hosts such as Sonic Visualiser.
The library is cross-platform and supports Linux, Mac OS X and Windows systems. Essentia is designed with a focus on the robustness of the provided music descriptors and is optimized in terms of the computational cost of the algorithms. The provided functionality, specifically the music descriptors included out-of-the-box and signal processing algorithms, is easily expandable and allows for both research experiments and development of large-scale industrial applications.
Essentia has been in development for more than 7 years incorporating the work of more than 20 researchers and
developers through its history. The 2.0 version marked the first release to be publicly available as free software released under AGPLv3.
developers through its history. The 2.0 version marked the first release to be publicly available as free software released under AGPLv3.
Algorithms
Essentia currently features the following algorithms (among others):
- Audio file input/output: ability to read and write nearly all audio file formats (wav, mp3, ogg, flac, etc.)
- Standard signal processing blocks: FFT, DCT, frame cutter, windowing, envelope, smoothing
- Filters (FIR & IIR): low/high/band pass, band reject, DC removal, equal loudness
- Statistical descriptors: median, mean, variance, power means, raw and central moments, spread, kurtosis, skewness, flatness
- Time-domain descriptors: duration, loudness, LARM, Leq, Vickers’ loudness, zero-crossing-rate, log attack time and other signal envelope descriptors
- Spectral descriptors: Bark/Mel/ERB bands, MFCC, GFCC, LPC, spectral peaks, complexity, rolloff, contrast, HFC, inharmonicity and dissonance
- Tonal descriptors: Pitch salience function, predominant melody and pitch, HPCP (chroma) related features, chords, key and scale, tuning frequency
- Rhythm descriptors: beat detection, BPM, onset detection, rhythm transform, beat loudness
- Other high-level descriptors: danceability, dynamic complexity, audio segmentation, semantic annotations based on SVM classifiers
The complete list of algorithms is available online in the official documentation.
Architecture
The main purpose of Essentia is to serve as a library of signal-processing blocks. As such, it is intended to provide as many algorithms as possible, while trying to be as little intrusive as possible. Each processing block is called an Algorithm, and it has three different types of attributes: inputs, outputs and parameters. Algorithms can be combined into more complex ones, which are also instances of the base Algorithm class and behave in the same way. An example of such a composite algorithm is presented in the figure below. It shows a composite tonal key/scale extractor, which combines the algorithms for frame cutting, windowing, spectrum computation, spectral peaks detection, chroma features (HPCP) computation and finally the algorithm for key/scale estimation from the HPCP (itself a composite algorithm).

The algorithms can be used in two different modes: standard and streaming. The standard mode is imperative while the streaming mode is declarative. The standard mode requires to specifying the inputs and outputs for each algorithm and calling their processing function explicitly. If the user wants to run a network of connected algorithms, he/she will need to manually run each algorithm. The advantage of this mode is that it allows very rapid prototyping (especially when the python bindings are coupled with a scientific environment in python, such as ipython, numpy, and matplotlib).
The streaming mode, on the other hand, allows to define a network of connected algorithms, and then an internal scheduler takes care of passing data between the algorithms inputs and outputs and calling the algorithms in the appropriate order. The scheduler available in Essentia is optimized for analysis tasks, and does not take into account the latency of the network. For real-time applications, one could easily replace this scheduler with another one that favors latency over throughput. The advantage of this mode is that it results in simpler and safer code (as the user only needs to create algorithms and connect them, there is no room for him to make mistakes in the execution order of the algorithms), and in lower memory consumption in general, as the data is streamed through the network instead of being loaded entirely in memory (which is the usual case when working with the standard mode). Even though most of the algorithms are available for both the standard and streaming mode, the code that implements them is not duplicated as either the streaming version of an algorithm is deduced/wrapped from its standard implementation, or vice versa.
Applications
Essentia has served in a large number of research activities conducted at Music Technology Group since 2006. It has been used for music classification, semantic autotagging, music similarity and recommendation, visualization and interaction with music, sound indexing, musical instruments detection, cover detection, beat detection, and acoustic analysis of stimuli for neuroimaging studies. Essentia and Gaia have been used extensively in a number of research projects and industrial applications. As an example, both libraries are employed for large-scale indexing and content-based search of sound recordings within Freesound, a popular repository of Creative Commons licensed audio samples. In particular, Freesound uses audio based similarity to recommend sounds similar to user queries. Dunya is a web-based software application using Essentia that lets users interact with an audio music collection through the use of musical concepts that are derived from a specific musical culture, in this case Carnatic music.
Examples
Essentia can be easily used via its python bindings. Below is a quick illustration of Essentia’s possibilities for example on detecting beat positions of music track and itspredominant melody in a few lines of python code using the standard mode:
from essentia.standard import *;
audio = MonoLoader(filename = 'audio.mp3')();
beats, bconfidence = BeatTrackerMultiFeature()(audio);
print beats;
audio = EqualLoudness()(audio);
melody, mconfidence = PredominantMelody(guessUnvoiced=True, frameSize=2048, hopSize=128)(audio);
print melody
Another python example for computation of MFCC
features using the streaming mode:
Vamp plugin provided with Essentia allows to use many of its algorithms via the graphical interface of Sonic Visualiser. In this example, positions of onsets are computed for a music piece (marked in red):

features using the streaming mode:
from essentia.streaming import *
loader = MonoLoader(filename = 'audio.mp3')
frameCutter = FrameCutter(frameSize = 1024, hopSize = 512)
w = Windowing(type = 'hann')
spectrum = Spectrum()
mfcc = MFCC()
pool = essentia.Pool()
# connect all algorithms into a network
loader.audio >> frameCutter.signal
frameCutter.frame >> w.frame >> spectrum.frame
spectrum.spectrum >> mfcc.spectrum
mfcc.mfcc >> (pool, 'mfcc')
mfcc.bands >> (pool, 'mfcc_bands')
# compute network
essentia.run(loader)
print pool['mfcc']
print pool['mfcc_bands']
Vamp plugin provided with Essentia allows to use many of its algorithms via the graphical interface of Sonic Visualiser. In this example, positions of onsets are computed for a music piece (marked in red):

An interested reader is referred to the documention online for more example applications built on top of Essentia.
Getting Essentia
The detailed information about Essentia is available online on the official web page: http://essentia.upf.edu. It contains the complete documentation for the project, compilation instructions for Debian/Ubuntu, Mac OS X and Windows, as well as precompiled packages. The source code is available at the official Github repository:http://github.com/MTG/essentia. In our current work we are focused on expanding the library and the community of users, and all active Essentia users are encouraged to contribute to the library.
References
[1] Serra, X., Magas, M., Benetos, E., Chudy, M., Dixon, S., Flexer, A., Gómez, E., Gouyon, F., Herrera, P., Jordà, S., Paytuvi, O, Peeters, G., Schlüter, J., Vinet, H., and Widmer, G., Roadmap for Music Information ReSearch, G. Peeters, Ed., 2013. [Online].
[2] Bogdanov, D., Wack N., Gómez E., Gulati S., Herrera P., Mayor O., Roma, G., Salamon, J., Zapata, J., Serra, X. (2013). ESSENTIA: an Audio Analysis Library for Music Information Retrieval. International Society for Music Information Retrieval Conference(ISMIR’13). 493-498.
[3] Bogdanov, D., Wack N., Gómez E., Gulati S., Herrera P., Mayor O., Roma, G., Salamon, J., Zapata, J., Serra, X. (2013). ESSENTIA: an Open-Source Library for Sound and Music Analysis. ACM International Conference on Multimedia (MM’13).
http://www.dkislyuk.com/posts/building-an-audio-classifier-part-2/
http://modelai.gettysburg.edu/2012/music/
MusicMood: A Machine Learning Approach to Classify Music by Mood Based on Song Lyrics
Written by: Sebastian Raschka
Primary Source: Sebastian Raschka
In this article, I want to share my experience with a recent data mining project which probably was one of my most favorite hobby projects so far. It’s all about building a classification model that can automatically predict the mood of music based on song lyrics.
Links
Sections
- About the Project
- Data Collection and Exploratory Analysis
- Model Selection and Training
- Deploying the Webapp
- Future Plans
About the Project
[back to top]
The goal of this project was to build a classifier that categorizes songs into happy and sad. As follow-up to my previous article Naive Bayes and Text Classification I – Introduction and Theory, I wanted to focus on the song lyrics only in order to build such a classification model ‒ a more detailed technical report is in progress ‒ and here, I want to share the experiences.
The goal of this project was to build a classifier that categorizes songs into happy and sad. As follow-up to my previous article Naive Bayes and Text Classification I – Introduction and Theory, I wanted to focus on the song lyrics only in order to build such a classification model ‒ a more detailed technical report is in progress ‒ and here, I want to share the experiences.
Data collection, pre-processing, and model training was all done in Python using Pandas, scikit-learn, h5py, and the Natural Language Toolkit ‒ a very smooth and seamless experience up to the point where I tried to deploy the web app powered by Flask, but more on that later.
In hope that it might be useful to others, I uploaded all the data and code to a public GitHub repository, and I hope that I provided enough descriptive comments to outline the workflow.
WHY I WAS INTERESTED IN THIS PARTICULAR PROJECT
I have always had a big passion for the “data science” field which is one of the reason why I ended up pursuing a PhD as computational biologist who solves problems in the fields of protein structure modeling and analysis. About a year ago, I had the pleasure to take a great course about research in statistical pattern recognition that really fascinated me. Since I really enjoy music (classic rock in particular) and always wanted to take a dive into Python’s web frameworks, this suddenly all came together.
Data Collection and Exploratory Analysis
[back to top]
When I was brainstorming ideas about this project, I had no idea if there were freely available datasets that I could use. I soon found literature about related projects were the authors used hand-labeled datasets for mood prediction. I couldn’t find a source for downloading those datasets though, and those datasets seemed to have too many mood labels for my taste anyway which I thought could have a negative impact on the predictive performance. For this project, I just wanted to focus on the two classes happy and sad, because I thought that a binary classification based on song lyrics only might already be challenging enough for a machine learning algorithm based on text analysis.
When I was brainstorming ideas about this project, I had no idea if there were freely available datasets that I could use. I soon found literature about related projects were the authors used hand-labeled datasets for mood prediction. I couldn’t find a source for downloading those datasets though, and those datasets seemed to have too many mood labels for my taste anyway which I thought could have a negative impact on the predictive performance. For this project, I just wanted to focus on the two classes happy and sad, because I thought that a binary classification based on song lyrics only might already be challenging enough for a machine learning algorithm based on text analysis.
THE MILLION SONG DATASET AND LYRICS
Next, I stumbled upon the Million Song Dataset, which I found quite interesting. There is also the related musiXmatch catalog which provides lyrics for the Million Song Dataset. However, the lyrics in musiXmatch are already pre-processed, and my plan was to compare different pre-processing techniques. Plus I thought that the creation of an own mood-labeled song lyrics dataset might be a good exercise anyway. So I wrote some simple scripts to download the lyrics from LyricsWiki, filtered out songs for which lyrics were not available, and automatically removed non-English songs using Python’s Natural Language Toolkit.
MOOD LABELS – WHERE TO GET THEM?
So far so good, now that I collected a bunch of songs and the accompanying lyrics, the next task was to get the mood labels. In my first attempt, I downloaded user-provided tags from Last.fm, but I soon found out that tags like happy and sad (and other related adjectives) were only available for a very small subset of songs and very, very contradictory since being incomplete or out of context. Thus, I decided to do it the hard way and hand-label a subset of 1200 songs: 1000 songs for the training dataset and 200 songs for the validation dataset. There is no question about it that associating music with a particular mood is a somewhat subjective task, and if the labels are provided by a single person only, it unarguably introduces another bias. But let me explain later in the Webapp section how I am planning to extend the dataset and want to deal with this bias.
LABELING DATA CAN ACTUALLY BE FUN
Eventually, I ended up listening to 1200 songs while reading the lyrics. Of course, this sounds very tedious, however, I have to say that I also enjoyed this task, since I discovered a lot of good and interesting songs during this process! I used the following guidelines to assign the happy and sad mood labels: If the song had a somewhat dark theme, e.g., violence, war, killing, etc. (unfortunately there were quite a few songs matching these criteria), I labeled it as sad. Also, if the artist seemed to be upset or complaining about something, or if the song was about a “lost love,” I also labeled it as sad. And basically everything else was labeled as happy.
EXPLORATORY VISUALIZATION TO SATISISFY CURIOSITY
After I finished labeling the 1000-song training dataset, I was really tempted to do some exploratory analysis and plotted the number of happy and sad songs over the years. I found the results really interesting: Although there is a large bias towards more recent releases in the Million Song Dataset, there seems to bea trend: Unfortunately, music seems to become sadder over the years.

Model Selection and Training
NAIVE BAYES – WHY?
As I mentioned in the introduction, one reason why I focused on naive Bayes classification for this project was to have an application for the previous Naive Bayes and Text Classification I – Introduction and Theory article. However, since I was also planning to create a small web app, I wanted to have a computationally efficient classifier. Some of the advantages of naive Bayes models are that they are pretty efficient to train in the batch-learning mode while they are also very compatible to on-line learning (i.e., updates on-the fly when new labeled data arrives). By the way, the predictive performance of naive Bayes classifiers is actually not too bad in context of text categorization. Studies showed that naive Bayes models tend to perform well given small sample sizes [1] and they are successfully being used for similar binary text classification tasks such as e-mail spam detection [2]. Other empirical studies have also shown that the performance of naive Bayes classifier for text categorization is comparable to support vector machines [3][4].
[1] P. Domingos and M. Pazzani. On the optimality of the simple bayesian classifier under zero-one loss. Machine learning, 29(2-3):103–130, 1997.
[2] M. Sahami, S. Dumais, D. Heckerman, and E. Horvitz. A bayesian approach to filtering junk e-mail. In Learning for Text Categorization: Papers from the 1998 workshop, volume 62, pages 98–105, 1998.
[3] S. Hassan, M. Rafi, and M. S. Shaikh. Comparing svm and naive bayes classifiers for text categorization with wikitology as knowledge enrichment. In Multitopic Conference (INMIC), 2011 IEEE 14th International, pages 31–34. IEEE, 2011.
[4] A. Go, R. Bhayani, and L. Huang. Twitter sentiment classification using distant supervision. CS224N Project Report, Stanford, pages 1–12, 2009.
[2] M. Sahami, S. Dumais, D. Heckerman, and E. Horvitz. A bayesian approach to filtering junk e-mail. In Learning for Text Categorization: Papers from the 1998 workshop, volume 62, pages 98–105, 1998.
[3] S. Hassan, M. Rafi, and M. S. Shaikh. Comparing svm and naive bayes classifiers for text categorization with wikitology as knowledge enrichment. In Multitopic Conference (INMIC), 2011 IEEE 14th International, pages 31–34. IEEE, 2011.
[4] A. Go, R. Bhayani, and L. Huang. Twitter sentiment classification using distant supervision. CS224N Project Report, Stanford, pages 1–12, 2009.
GRID SEARCH AND THE FINAL MODEL
I especially want to highlight the great GridSearch implementation in scikit-learn that made the search for the “optimal” combination between pre-processing steps and estimator parameters very convenient. Here, I focussed on optimizing precision and recall via the F1-score performance metric rather than optimizing the overall accuracy ‒ I was primarily interested in filtering out sad songs; one might argue that this could be an interesting application to remove all the sad stuff from one’s music library.
I don’t want to go into too much detail about the model selection in this article (since this will be part of a separate report), so I will just provide a very brief overview of the final model choice: The combination of 1-gram tokenization, stop word removal and porter stemming as well as feature normalization via term frequency-inverse document frequencyin combination with a multinomial naive Bayes model seemed work best (in terms of the F1-score). However, the differences between the different pre-processing steps and parameter choices were rather minor except for the choice of the n-gram sequence lengths:


Deploying the Web app
[back to top]
I was particularly looking forward to part about turning the the final classifier into a web app. I have never done this before, and I was really eager to dive into Django or Flask. After browsing through some introductory tutorials, I decided to go with Flask, because being more lightweight it seemed to be a little bit more appropriate for this task. Embedding the classifier into a Flask web framework was actually way more straightforward than I initially thought ‒ Flask is just such a nice library and really easy to learn!
I was particularly looking forward to part about turning the the final classifier into a web app. I have never done this before, and I was really eager to dive into Django or Flask. After browsing through some introductory tutorials, I decided to go with Flask, because being more lightweight it seemed to be a little bit more appropriate for this task. Embedding the classifier into a Flask web framework was actually way more straightforward than I initially thought ‒ Flask is just such a nice library and really easy to learn!
THE MAGIC NUMBER 500
However, the hard part (and probably the most challenging part of this whole project) was actually to deploy the app on a web server. The problems started when I set up a new Python environment on my bluehost server (I have a “starter” shared hosting plan). After I eventually got all the C-extensions compiled and installed “properly,” there was another thing to deal with in order to make sure that the Apache server digests my code: FastCGI. Luckily, there was this nice Flask tutorial to also overcome this challenge. Okay, theoretically I was all set ‒ at least I thought so. When I tried to use the web online for the first time, I remember that everything worked fine. Nice, after I tested the Flask app locally, the app also seemed to work on the web server! Unfortunately, though, the initial joy of the work didn’t last very long when I saw the server throwing “500 Internal Server Error”s once in a while (or rather 80% of the time). I literally worked through hundreds of troubleshooting guides and couldn’t find any reason why. In this particular case, no error message was written to the error log, which was different, for example, when I provoked other 500 errors intentionally.
PYTHONANYWHERE TO THE RESCUE
Eventually, I narrowed it down to the parts of the code where scikit-learn/scipy/numpy code was executed. Thus, my conclusion was that something with the C-libs probably caused the hiccups on this particular server platform. I also contacted the help staff and scripters at bluehost, but, unfortunately, they also couldn’t tell me anything about the particular cause of this issue. At this point, I was pretty much frustrated, since I put a lot of effort into something that didn’t seem to work for unexplained reasons. Still being curious if there was a general issue with my code, I just signed up for a free account at pythonanywhere ‒ and see what happened: Without having to install any additional Python libraries, my app just worked magically. However, I will probably try out some other things on the bluehost server in future, snce I don’t like this sort of “unfinished business,” and I am really looking forward to any sort of tips and suggestions that could help with resolving this issue.
As you can see, although I used a 10-fold cross-validation approach in the modeling selection process the model is still quite prone to over-fitting. The rather small training dataset might be one of the factors, which can hopefully be overcome in future
Future Plans
ARE THERE ANY PLANS TO UPDATE THE CLASSIFIER?
Yes! My initial plan was to implement the naive Bayes classifier in an on-line learning mode so that it will be updated every time a user provides feedback about a classification. However, my feeling is that there will be a growing bias towards a certain subset of the most popular contemporary songs. Because I am also very eager to extend the training dataset for other analyses, e.g., comparisons of different machine learning algorithms and performance comparisons with regard to different training dataset sizes, I opted for another solution. Right now, I save the songs, lyrics, and suggested mood labels to a database if a user provides voluntary feedback about the prediction. After certain time intervals, I am planning to re-train and re-evaluate the model to hopefully improve the predictive performance and gain some interesting insights. The mood label assignment is highly subjective of course, thus, I am saving multiple mood labels per song to the database so that the “ground truth” label can be determined by majority rule. Also, this can open a door to some interesting regression-based analysis.
WHAT ABOUT SOUND DATA?
I thought about including sound data in the classification. However, I think the challenge is that sound data is hard to obtain. Sure, there are those HDF5 files in the Million Song Dataset with pre-extracted sound features, but what about new songs that are not in the training dataset? Maybe data streaming from YouTube could be a possibility to be explored in future.

I am a PhD student at Michigan State University and departmental representative in the Council of Graduate Students. Based on groundbreaking concept, I am currently developing a novel drug screening software in theProtein Structural Analysis lab.
SoundRecordingsClassification
example showing classification of sound recordings
Data
Test sound
Making test sound with variable amplitude and frequency in audacity 

Magnatagatune
Dataset of music excerpts labeled by TagATune game players. Analysis of structure are also possible by EchoNest.
- magnatagatune
- downloaded (without recordings)
Xeno-canto
Dataset of birds song recordings with annotations.
- http://www.xeno-canto.org/
- automatic downloading script
Signal processing
Processing recordings into feature vectors.
- MATLAB / GNU Octave
- agentOctave manual
- (agentOctave at aistorm (svn))
- extracting standard deviation for volume representation, max power frequency and zero crossing rate for information about frequency
- Python
Vector quantization
- making codebook vector for all recordings using k-means
- setting code vector for each recording
- Test sound with 10 frames per second
- FFT has 10Hz resolution 44,1 kHz sample rate
- and 3 clusters in VQ

- Test sound with 100 frames per second
- FFT has 100Hz resolution 44,1 kHz sample rate
- and 2 clusters in VQ

Algorithms
Clustering
- Algorithms for time series comparison
- MATLAB: Self-organizing map
- Weka
- k-means bash script
- EM (using GUI)
- SOM (using GUI)
- Mappa: SOM in C++
- SOM bash script
Classification
- Time series classification using k-Nearest neighbours, Multilayer Perceptronand Learning Vector Quantization algorithms
- Octave: Multi-Layer Perceptron
- Weka
- k-NN
- Mappa: LVQ in C++
- LVQ bash script
Semi-supervised learning
Hidden Markov Models
Dynamic Time Warping
Results comparison
- matching matrix bash script:
- confussion matrix bash script
https://code.google.com/p/soundpylab/wiki/SoundRecordingsClassification
Music software written in Python
Audio Players
- Bluemindo - Bluemindo is a really simple but powerful audio player in Python/PyGTK, using Gstreamer. Bluemindo is a free (as in freedom) software, released under GPLv3, only.
- cplay - a curses front-end for various audio players
- edna - an MP3 server, edna allows you to access your MP3 collection from any networked computer. The web pages are dynamically constructed, adjusting to directory structure and the files in those directories. This is much nicer than using simple directory indexing. Rather than directly serving up an MP3, the software serves up a playlist. This gets passed to your player (e.g. WinAmp) which turns around with an HTTP request to stream the MP3.
- Listen - Music management and playback for GNOME
- MediaCore Audio/Podcast Player and CMS - Web based CMS for music management in video, audio and podcast form. All audio, video, and podcasts added to the system are playable from any browser.
- MMA - Musical Midi Accompaniment. If you follow the above link you will find that Pymprovisator is no longer developed due to the fact that there is this similar, but more powerful GPL Python software.
- Peyote - Peyote is an audio player with friendly MC-like interface. Peyote is designed specifically for work easy with cue sheets.
- Pymprovisator - Pymprovisator is a program that emulates the program Band in a Box from PG Music. You can think in it like the electronic version of the books+CD from Jamey Aebersold. You set the basic parameters in a song: title, style, key, chords sequence,... and the program will generate a Midi file with the correct accompaniment. (dev suspended)
- Pymps - Pymps is the PYthon Music Playing System - a web based mp3/ogg jukebox. It's written in Python and utilises the PostgreSQL database.
- MusicPlayer - MusicPlayer is a high-quality music player implemented in Python, using FFmpeg and PortAudio.
- Pymserv - PyMServ is a graphical client for mserv, a music server. It is written in Python using pygtk and gconf to store prefs.
- Quod Libet - Quod Libet is a GTK+-based audio player written in Python. It lets you make playlists based on regular expressions. It lets you display and edit any tags you want in the file. And it lets you do this for all the file formats it supports -- Ogg Vorbis, FLAC, MP3, Musepack, and MOD.
- TheTurcanator - a small midi piano tutor for windows and mac. Includes CoreMIDI wrapper written in pyrex.
Audio Convertors
- audio-convert-mod - audio-convert-mod is a simple audio file converter that supports many formats. At just a right-click, you can convert any amount of music files to WAV, MP3, AAC, Ogg and more. audio-convert-mod was designed with the same principles as fwbackups - keeping things simple.
- SoundConverter - SoundConverter is a simple audio file converter for the GNOME desktop, using GStreamer for conversion. It can read anything GStreamer has support for, and writes to WAV, MP3, AAC, Ogg or FLAC files.
- Python Audio Tools - Python audio tools are a collection of audio handling programs which work from the command line. These include programs for CD extraction, track conversion from one audio format to another, track renaming and retagging, track identification, CD burning from tracks, and more. Supports internationalized track filenames and metadata using Unicode. Works with high-definition, multi-channel audio as well as CD-quality. Track conversion uses multiple CPUs or CPU cores if available to greatly speed the transcoding process. Track metadata can be retrieved from FreeDB, MusicBrainz or compatible servers. Audio formats supported are: WAV, AIFF, FLAC, Apple Lossless, Shorten, WavPack, MP3, MP2, M4A, Ogg Vorbis, Ogg Speex, Ogg FLAC, & Sun AU
Music Notation
- Abjad - Abjad is a Python API for Formalized Score Control. Abjad is designed to help composers build up complex pieces of music notation in an iterative and incremental way. You can use Abjad to create a symbolic representation of all the notes, rests, staves, nested rhythms, beams, slurs and other notational elements in any score. Because Abjad wraps the powerful LilyPond music notation package, you can use Abjad to control extremely fine-grained typographic details of all elements of any score.
- Frescobaldi - is a LilyPond music score editor written in Python using PyQt4 and PyKDE4. Clicking a button runs LilyPond on the current document and displays the PDF in a preview window. There are some nice editing tools and a powerful score wizard to quickly setup a template score.
- mingus - mingus is an advanced music theory and notation package for Python. It can be used to play around with music theory, to build editors, educational tools and other applications that need to process music. It can also be used to create sheet music with LilyPond and do automated musicological analysis.
- see also 'music21' below
Musical Analysis
- music21 - a toolkit developed at MIT for computational musicology, music theory, and generative composition. Provides expandable objects and methods for most common theoretical problems. Supports music import via MusicXML, Humdrum/Kern, Musedata, ABC, and MIDI, output via MusicXML, Lilypond, and MIDI, and can easily integrate with notation editors (Finale, Sibelius, or MuseScore) and other audio and DAW software (via MIDI).
- pcsets - Pitch Class Sets are a mathematical model for analyzing and composing music.
- PyOracle - Module for Audio Oracle and Factor Oracle Musical Analysis.
Audio Analysis
- Friture - Friture is a graphical program designed to do time-frequency analysis on audio input in real-time. It provides a set of visualization widgets to display audio data, such as a scope, a spectrum analyser, a rolling 2D spectrogram.
- Yaafe - Yet Another Audio Feature Extractor is a toolbox for audio analysis. Easy to use and efficient at extracting a large number of audio features simultaneously. WAV and MP3 files supported, or embedding in C++, Python or Matlab applications.
- Aubio - Aubio is a tool designed for the extraction of annotations from audio signals. Its features include segmenting a sound file before each of its attacks, performing pitch detection, tapping the beat and producing midi streams from live audio.
- LibROSA - A python module for audio and music analysis. It is easy to use, and implements many commonly used features for music analysis.
Ear Training
- GNU Solfege - GNU Solfege is a computer program written to help you practice ear training. It can be useful when practicing the simple and mechanical exercises.
cSound
- athenaCL - modular, polyphonic, poly-paradigm algorithmic music composition in an interactive command-line environment. The athenaCL system is an open-source, cross-platform, object-oriented composition tool written in Python; it can be scripted and embedded, includes integrated instrument libraries, post-tonal and microtonal pitch modeling tools, multiple-format graphical outputs, and musical output in Csound, MIDI, audio file, XML, and text formats.
- Cabel - Visual way to create csound instruments.
- Dex Tracker - Front end for csound that includes a tracker style score editor in a grid, text editor, cabel tested with Python 2.5.
- Ounk is a Python audio scripting environment that uses Csound as it's engine.
- Cecilia is a csound frontend that lets you create your own GUI (grapher, sliders, toggles, popup menus) using a simple syntax. Cecilia comes with a lots of original builtin modules for sound effects and synthesis. Previously written in tcl/tk, Cecilia was entirely rewritten with Python/wxPython and uses the Csound API for communicating between the interface and the audio engine. Version 4.02 beta is the current release.
- see also 'blue' below
Audio (Visual) Programming Frameworks
- Peace Synthesizer Framework - "Peace Synthesizer Framework" is Cross Platform Scriptable Real-Time Visualization & Sound. It has internal and external real-time scriptable visualization and sound generation and also support Nintendo system [Famicom] - like sound Emulation for 8-bits style chiptune music.
- Hypersonic - Hypersonic is for building and manipulating sound processing pipelines. It is designed for real-time control. It includes objects for oscillators, filters, file-io, soundcard and memory operations.
Music programming in Python
Playing & creating sound
- pydub - Pydub is a simple and easy high level interface based on ffmpeg and influenced by jquery. It manipulates audio, adding effects, id3 tags, slicing, concatenating audio tracks. Supports python 2.6, 2.7, 3.2, 3.3
- audiere - Audiere is a high-level audio API. It can play Ogg VorbisAU, MP3, FLACAS, uncompressed WAV, AIFF, MOD, S3M, XM, and ITAN files. For audio output, Audiere supports DirectSound or WinMM in Windows, OSS on Linux and Cygwin, and SGI AL on IRIX.
- audiolab - audiolab is a small Python package (now part of scikits) to import data from audio files to numpy arrays and export data from numpy arrays to audio files. It uses libsndfile from Erik Castro de Lopo for the underlying IO, which supports many different audio formats: http://www.mega-nerd.com/libsndfile/
- GStreamer Python Bindings - GStreamer is a big multimedia library, it is very simple to use it with these python bindings. Many applications rely on it (Exaile, Pitivi, Jokosher, Listen usw.). Online documentation can be found on http://pygstdocs.berlios.de/
- improviser - Automatic music generation software. Experiments in musical content generation.
- python-musical - Python library for music theory, synthesis, and playback. Contains a collection of audio wave generators and filters powered by numpy. Also contains a pythonic music theory library for handling notes, chords, scales. Can load, save, and playback audio.
- LoopJam - Instant 1 click remixing of sample loops, able to boost your creativity and multiply your sample loop library. Remix audio loops on a slice level, apply up to 9 FX to individual slices or create countless versions using LJ's auto-remix feature (jam) which re-arranges the audio loop forming musical patterns.
- Loris - Loris is an Open Source C++ class library implementing analysis, manipulation, and synthesis of digitized sounds using the Reassigned Bandwidth-Enhanced Additive Sound Model. Loris supports modified resynthesis and manipulations of the model data, such as time- and frequency-scale modification and sound morphing. Loris includes support and wrapper code for building extension modules for various scripting languages (Python, Tcl, Perl).
- MusicKit - The MusicKit is an object-oriented software system for building music, sound, signal processing, and MIDI applications. It has been used in such diverse commercial applications as music sequencers, computer games, and document processors. Professors and students in academia have used the MusicKit in a host of areas, including music performance, scientific experiments, computer-aided instruction, and physical modeling. PyObjC is required to use this library in Python.
- pyao - pyao provides Python bindings for libao, a cross-platform audio output library. It supports audio output on Linux (OSS, ALSA, PulseAudio, esd), MacOS X, Windows, *BSD and some more. There are ready-to-use packages in Debian/Ubuntu, and Audio output is as easy as:import ao; pcm = ao.AudioDevice("pulse"); pcm.play(data)
- pyFluidSynth - Python bindings for FluidSynth, a MIDI synthesizer that uses SoundFont instruments. This module contains Python bindings for FluidSynth.FluidSynth is a software synthesizer for generating music. It works like a MIDI synthesizer. You load patches, set parameters, then send NOTEON and NOTEOFF events to play notes. Instruments are defined in SoundFonts, generally files with the extension SF2. FluidSynth can either be used to play audio itself, or you can call a function that returns chunks of audio data and output the data to the soundcard yourself.
- Pygame - Pygame is a set of Python modules designed for writing games. It is written on top of the excellent SDL library. This allows you to create fully featured games and multimedia programs in the Python language. Pygame is highly portable and runs on nearly every platform and operating system. .ogg .wav .midi .mod .xm .mp3. Sound output. midi input and output. Load sounds into numeric and numpy arrays.
- PyMedia - (Not updated since 2006) PyMedia is a Python module for the multimedia purposes. It provides rich and simple interface for the digital media manipulation( wav, mp3, ogg, avi, divx, dvd, cdda etc ). It includes parsing, demutiplexing, multiplexing, coding and decoding. It can be compiled for Windows, Linux and cygwin.
- pyo - pyo is a Python module containing classes for a wide variety of audio signal processing types. With pyo, user will be able to include signal processing chains directly in Python scripts or projects, and to manipulate them in real time through the interpreter. Tools in pyo module offer primitives, like mathematical operations on audio signal, basic signal processing (filters, delays, synthesis generators, etc.), but also complex algorithms to create sound granulation and others creative audio manipulations. pyo supports OSC protocol (Open Sound Control), to ease communications between softwares, and MIDI protocol, for generating sound events and controlling process parameters. pyo allows creation of sophisticated signal processing chains with all the benefits of a mature, and wildly used, general programming language.
- Zyne - Zyne is a Python modular synthesizer using pyo as its audio engine. Zyne comes with more than 10 builtin modules implementing different kind of synthesis engines and provides a simple API to create your own custom modules.
- Soundgrain - Soundgrain is a graphical interface where users can draw and edit trajectories to control granular sound synthesis modules. Soundgrain is written with Python and WxPython and uses pyo as its audio engine.
- pySonic - (Not updated since 2005) pySonic is a Python wrapper around the high performance, cross platform, but closed source, FMOD sound library. You get all the benefits of the FMOD library, but in a Pythonic, object oriented package.
- PySndObj - The Sound Object Library is an object-oriented audio processing library. It provides objects for synthesis and processing of sound that can be used to build applications for computer-generated music. The core code, including soundfile and text input/output, is fully portable across several platforms. Platform-specific code includes realtime audio IO and MIDI input support for Linux (OSS,ALSA and Jack), Windows (MME and ASIO), MacOS X (CoreAudio, but no MIDI at moment), Silicon Graphics (Irix) machines and any Open Sound System-supported UNIX. The SndObj library also exists as Python module, aka PySndObj. The programming principles for Python SndObj programming are similar to the ones used in C++. It is also possible to use the Python interpreter for on-the-fly synthesis programming.
- PySynth - A simple music synthesizer.
- Snack - (last update: December 2005) The Snack Sound Toolkit is designed to be used with a scripting language such as Tcl/Tk or Python. Using Snack you can create powerful multi-platform audio applications with just a few lines of code. Snack has commands for basic sound handling, such as playback, recording, file and socket I/O. Snack also provides primitives for sound visualization, e.g. waveforms and spectrograms. It was developed mainly to handle digital recordings of speech (being developped at the KTH music&speech department), but is just as useful for general audio. Snack has also successfully been applied to other one-dimensional signals. The combination of Snack and a scripting language makes it possible to create sound tools and applications with a minimum of effort. This is due to the rapid development nature of scripting languages. As a bonus you get an application that is cross-platform from start. It is also easy to integrate Snack based applications with existing sound analysis software.
- AudioLazy - Real-Time Expressive Digital Signal Processing (DSP) Package for Python, using any Python iterable as a [-1;1] range audio source. Has time-variant linear filters as well as LTI filters using Z-Transform equations like 1 - z ** -1, as well as analysis (ZCR / zero crossing rate, LPC / Linear Predictive Coding, AMDF, etc.), synthesis (table lookup, ADSR, etc.), ear modeling (Patterson-Holdsworth with gammatone filters and ERB models), and multiple implementation of common filters (lowpass, highpass, comb, resonator), among several other resources (e.g. Lagrange polynomial interpolation, simple converters among MIDI pitch / frequency / string). Works mainly with Stream instances for its signal outputs, a generator-like (lazy) iterable with elementwise/broadcast-style operators similar to the Numpy array operators. Integrated with Matplotlib for LTI filter plotting, although it doesn't require Matplotlib nor Numpy for computation, DSP or I/O. Emphasizes sample-based processing while keeping block-based processing easy to be done, this package can also be seen as a highly enhanced itertools. Pure Python, multiplatform, compatible with Python 2.7 and 3.2+, uses PyAudio for audio I/O (if needed). Can be used together with Scipy, Sympy, music21 and several other packages, none required for DSP computation based on Python iterables.
Community
- PythonSound - The Python Sound Project aims to develop a productive community around Python, Csound and other synthesis engines as tools for algorithmic and computer assisted composition of electroacoustic music.
Csound
- CSound / CsoundAC - Csound is a sound and music synthesis system, providing facilities for composition and performance over a wide range of platforms and for any style of music. The Csound orchestra language features over 1200 unit generators (called "opcodes") covering nearly every sound synthesis method and that the user can combine into "instruments" of unlimited complexity and flexibility. Csound 5 allows Python code to be called from or directly embedded into Csound orchestras. Additionally, the csnd Python extension module wraps the Csound API so that Csound can be embedded into Python applications. CsoundAC (for "Csound Algorithmic Composition") is a GUI front end to Csound with Python scripting and a Python module providing tools for the algorithmic generation or manipulation of Csound scores. Csound on Sourceforge; Csound-Python and Csound (some brief tutorials on the OLPC Wiki)
- Csound Routines - set of routines to manipulate and convert csound files
- PMask - Python implementation of CMask, a stochastic event generator for Csound.
MP3 stuff and Metadata editors
- eyed3 - eyeD3 is a Python module and program for processing ID3 tags. Information about mp3 files (i.e bit rate, sample frequency, play time, etc.) is also provided. The formats supported are ID3 v1.0/v1.1 and v2.3/v2.4.
- mutagen - Mutagen is a Python module to handle audio metadata. It supports ASF, FLAC, M4A, Monkey's Audio, MP3, Musepack, Ogg FLAC, Ogg Speex, Ogg Theora, Ogg Vorbis, True Audio, WavPack and OptimFROG audio files. All versions of ID3v2 are supported, and all standard ID3v2.4 frames are parsed. It can read Xing headers to accurately calculate the bitrate and length of MP3s. ID3 and APEv2 tags can be edited regardless of audio format. It can also manipulate Ogg streams on an individual packet/page level.
- ID3.py - This module allows one to read and manipulate so-called ID3 informational tags on MP3 files through an object-oriented Python interface.
- id3reader.py - Id3reader.py is a Python module that reads ID3 metadata tags in MP3 files. It can read ID3v1, ID3v2.2, ID3v2.3, or ID3v2.4 tags. It does not write tags at all.
- mpgedit - mpgedit is an MPEG 1 layer 1/2/3 (mp3), MPEG 2, and MPEG 2.5 audio file editor that is capable of processing both Constant Bit Rate (CBR) and Variable Bit Rate (VBR) encoded files. mpgedit can cut an input MPEG file into one or more output files, as well as join one or more input MPEG files into a single output file. Since no file decoding / encoding occurs during editing, there is no audio quality loss when editing with mpgedit. A Python development toolkit enables Python developers to utilize the core mpgedit API, providing access to mp3 file playback, editing and indexing functionality.
- m3ute2 - m3ute2 is program for copying, moving, and otherwise organizing M3U playlists and directories. m3ute2 can also generate detailed reports about lists of files.
- mmpython - MMPython is a Media Meta Data retrieval framework. It retrieves metadata from mp3, ogg, avi, jpg, tiff and other file formats. Among others it thereby parses ID3v2, ID3v1, EXIF, IPTC and Vorbis data into an object oriented struture.
- KaaMetadata Sucessor of MMPython.
- PyID3 - pyid3 is a pure Python library for reading and writing id3 tags (version 1.0, 1.1, 2.3, 2.4, readonly support for 2.2). What makes this better than all the others? Testing! This library has been tested against some 200+ MB of just tags.
- beets - music tag correction and cataloging tool. Consists of both a command-line interface for music manipulation and a library for building related tools. Can automatically correct tags using the MusicBrainz database.
- see also: PySonic for programmable MP3 playback
MIDI Mania
- pygame.midi - is a portmidi wrapper orginally based on the pyportmidi wrapper. Also pygame.music can play midi files. Can get input from midi devices and can output to midi devices. For osx, linux and windows. New with pygame 1.9.0. python -m pygame.examples.midi --output
- pyMIDI - Provides object oriented programmatic manipulation of MIDI streams. Using this framework, you can read MIDI files from disk, build new MIDI streams, process, or filter preexisting streams, and write your changes back to disk. If you install this package on a Linux platform with alsalib, you can take advantage of the ALSA kernel sequencer, which provides low latency scheduling and receiving of MIDI events. SWIG is required to compile the ALSA extension sequencer extension. Although OS-X and Windows provide similar sequencer facilities, the current version of the API does not yet support them. Some buggs are remaining in this package (for example when trying to delete a track), it has not been updated since 2006. This package is by Giles Hall. A sourceforge download.
- midi.py - (DEAD LINK) - Python MIDI classes: meaningful data structures that represent MIDI events and other objects. You can read MIDI files to create such objects, or generate a collection of objects and use them to write a MIDI file.
- MIDI.py - This module offers functions: concatenate_scores(), grep(), merge_scores(), mix_scores(), midi2opus(), midi2score(), opus2midi(), opus2score(), play_score(), score2midi(), score2opus(), score2stats(), score_type(), segment(), timeshift() and to_millisecs(). Uses Python3. There is a call-compatible Lua module.
- PMIDI - The PMIDI library allows the generation of short MIDI sequences in Python code.The interface allows a programmer to specify songs, instruments, measures, and notes. Playback is handled by the Windows MIDI stream API so proper playback timing is handled by the OS rather than by client code. The library is especially useful for generating earcons.
- portmidizero - portmidizero is a simple ctypes wrapper for PortMidi in pure Python.
- PyChoReLib - Python Chord Recognition Library. This is a library that implements the transformation from a list of notenames to a chord name. The system can be taught new chords by example: tell it that ['c', 'e', 'g'] is called a 'C' chord, and using its built-in music knowledge it immediately recognizes all major triads in all keys and all inversions/permutations. Comes with a real-time midi-input demo program (needs PyPortMidi).
- PyMIDI - The MIDI module provides MIDI input parsers for Python. Package not updated since 2000.
- PyPortMidi - PyPortMidi is a Python wrapper for PortMidi. PortMidi is a cross-platform C library for realtime MIDI control. Using PyPortMidi, you can send and receive MIDI data in realtime from Python. Besides using PyPortMidi to communicate to synthesizers and the like, it is possible to use PyPortMidi as a way to send MIDI messages between software packages on the same computer. PyPortMidi is now maintained at http://bitbucket.org/aalex/pyportmidi/
- PythonMIDI - The Python Midi package is a collection of classes handling Midi in and output in the Python programming language.
- PySeq - Python bindings for ALSA using ctypes
- milk - Superceding the older Nam, milk provides Python with classes representing key MIDI sequencer components: MIDI I/O, EventLists, Plugins and a realtime Flow class. The components can be freely interconnected in a fashion very similar to physical MIDI cabling, however the milk event system is not limited to MIDI events alone; you can define your own extensions should the need arise. Website says it is unpolished and unfinished.
- pyrtmidi - rtmidi provides realtime MIDI input/output across Linux (ALSA), Macintosh OS X, SGI, and Windows (Multimedia Library) operating systems. It is very fast, has a clean and pythonic interface, and supports virtual ports, according to author Patrick Kidd. In fact it is a wrapper for Gary Scavone's rtmidi from here, rather than the address on this website:
- rtmidi-python - Another RtMidi wrapper.
- winmidi.pyd - A demo? of a Python extension interfacing to the native windows midi libs that developed from earlier attempts.
- win32midi - Some Python samples to demonstrate how to output MIDI stream on MS windows platform. Unlike previous links, these samples playback MIDI by directly calling the Win32 MIDI APIs without an intermediate portable library. It provides a simple player class for playing with MIDI sound using the synthesizer on the soundcard/onboard soundchip. A sample script is provided for testing it out. As it is still a work in progress, bugs are expected.
- midiutil - A pure Python library for generating Midi files
- Pyknon - Pyknon is a simple music library for Python hackers. With Pyknon you can generate Midi files quickly and reason about musical proprieties.
- Desfonema Sequencer - A tracker minded MIDI sequencer for Linux (ALSA) written in Python/PyGTK
- python-music-gen - Simple library to generate midi patterns from numbers. Useful for building generative music tools.
Other protocols
- OSC.py - Python classes for OpenSoundControl library client functionality. The OSC homepage is at http://opensoundcontrol.org
- Twisted-osc - OSC Library for Twisted, an event-driven Python framework. It could really be ported to a non-Twisted framework as well, but is currently in the process of possibly become an official part of Twisted.
- aiosc - Minimalistic OSC communication module using asyncio.
- pyalsaaudio - This package contains wrappers for accessing the ALSA API (The Advanced Linux Sound Architecture (ALSA) provides audio and MIDI functionality to the Linux operating system) from Python. It is fairly complete for PCM devices and Mixer access.
- pkaudio - pkaudio is a collection of Python-based modules for midi input, osc communication with supercollider, and pyqt functionality.
- PyJack - This is a Python C extension module which provides an interface to the Jack Audio Server. It is possible to access the Jack graph to perform port connections/disconnections, monitor graph change events, and to perform realtime audio capture and playback using Numeric Python arrays. This is released under the GPL.
MAX/MSP & PureData
- mxdublin - mxdublin is an object oriented framework to generate events in pd and max. pd, short for Pure Data, a graphical Computer Music System written by Miller S. Puckette. mxdublin is a real time Python user environment working within pd/max. It is designed to put logic into a sequence of events. Python has been chosen has the interface language to build and run sequencing objects. Has a prerequisites, the users needs to know a minimal of Python and pd/max.
- net.loadbang.jython is a package which supports the Python scripting/programming language within MXJ for Max/MSP. We use the Jython interpreter, which allows Python and Java to interact, and gives Python access to the standard Java libraries (as well as any other Java code available to MXJ).
- OpenExposition - OpenExposition is a library aimed at automatic generation of user interfaces. The programmer only needs to specify what parts of the application need to be exposed to the user, and OpenExposition does the rest. At present, OpenExposition allows access to variables (either directly or through a pair of set/get methods), and class methods. It can construct the user interface graphically (using either the multi-platform FLTK library or Cocoa on Mac OS X), programatically (through Python), aurally (using the speech synthesis and recognition capabilities on Mac OS X), and by building MAX/MSP externals that can then be used in MAX/MSP.
- Py/pyext - Python script objects is an object library providing a full integration of the Python scripting language into the PD (and in the future Max/MSP) real-time system. With the py object you can load Python modules and execute the functions therein. With pyext you can use Python classes to represent full-featured pd/Max message objects. Multithreading (detached methods) is supported for both objects. You can send messages to named objects or receive (with pyext) with Python methods.
- Purity is a Python library for Pure Data dynamic patching. The idea is to be able to harness the power of Pure Data for audio programming without having to use its graphical interface. Python's clear and intuitive syntax can be used with profit in order to create intricate patches with advanced string handling, graphical user interfaces and asynchronous network operations. Purity uses Twisted, an event-driven Python framework.
Music software supporting Python
Multitrack Studios
- REAPER - "Audio Production Without Limits": REAPER is a professional digital audio workstation (DAW) for Windows, OS X and WINE. It comes with an uncrippled evaluation licence and supports advanced audio and MIDI recording, arranging and mixing. The support of several plugin formats (like VST, DX and AU) as well as the extremely flexible routing capabilities make it a powerful production suite. Since version 3.12 REAPER is scriptable with Python, allowing access to internal actions and parts of the API.
- Ableton Live - Award-winning commercial music creation, production and performance platform for Mac OS and Windows. Live is far and away one of the most interesting and groundbreaking audio recording and sequencing tools to come along in the past five years. Live uses Python internally and an experimental API has been exposed at this site, and there is a discussion group here.
- blue - blue is a Java program for use with Csound. It's interface is much like a digital multitrack, but differs in that there timelines within timelines (polyObjects). This allows for a compositional organization in time that seems to me to be very intuitive, informative, and flexible. soundObjects are the building blocks within blue's score timeline. soundObjects can be lists of notes, algorithmic generators, Python script code, csound instrument definitions, and whatever plugins that are developed for blue. these soundObjects may be text based, but they can be completely GUI based as well.
- Jokosher - Jokosher is a simple yet powerful multi-track studio. With it you can create and record music, podcasts and more, all from an integrated simple environment. Jokosher is written in Python and uses the GNOME platform and the GTK widget set. The audio engine is powered by GStreamer, and we use Cairo for some of the graphics.
- Jeskola Buzz Modular - Buzz is a modular audio host that saw the beginning of it's development in 1997 leading the way in open plugin-format hosting (pre-VST) and a unique spin on modular routing using modules called Machines in the form of Generators, Effects, Control machines. Buzz's implementation of Python comes through the use of the Control plugin called PyBuzz, a fully customizable and assignable meta-editor created by Leonard Ritter (creator of the Lunar audio library and the Linux modular host, Aldrin). A discussion group can be found here
- PyDAW - PyDAW is a powerful pattern-based DAW and plugin suite for producing electronic music. The UI is written entirely in Python/PyQt, and the audio engine in C.
No comments:
Post a Comment